Hierarchical Motion Planning Framework for Realising Long-horizon Task
Having robots collaborating alongside humans and assisting them in daily life activities, such as tidying up, is extremely challenging.
Robots need to reason about how to split such complex tasks in to simpler subtasks, while being able to safely and robustly accomplish each of the individual subtasks. For instance, picking up the target object, then moving it to the front of the shelf, opening the shelf door, and finally successfully placing the object inside the shelf. Additionally, robots also need to be able to adapt their movements to unpredictable and dynamic changes, such as humans moving around, and to unexpected object properties, including the object turning out to be heavier or softer than anticipated.
In this project, in collaboration with Waseda University in Japan and under the auspices of The Alan Turing Institute, we develop learning and motion planning methods for realising long-horizon tasks that are adaptable and generalisable to objects of various different properties.
We use the example of stacking several boxes with different hidden properties, such as mass or even the contents, that require the robot to change the high level (long-horizon) plan, as well as the robot motion itself -- such as how fast it should move.
This project directly contributes to the Goal 3 of the Moonshot programme: Realisation of AI robots that autonomously learn, adapt to their environment, evolve in intelligence and act alongside human beings, by 2050.
For more information on the Goal 3 of the Moonshot programme check: Moonshot Programme Goal 3
Project Collaboration Timeline: First Phase (April 1, 2024 to November 30, 2025)
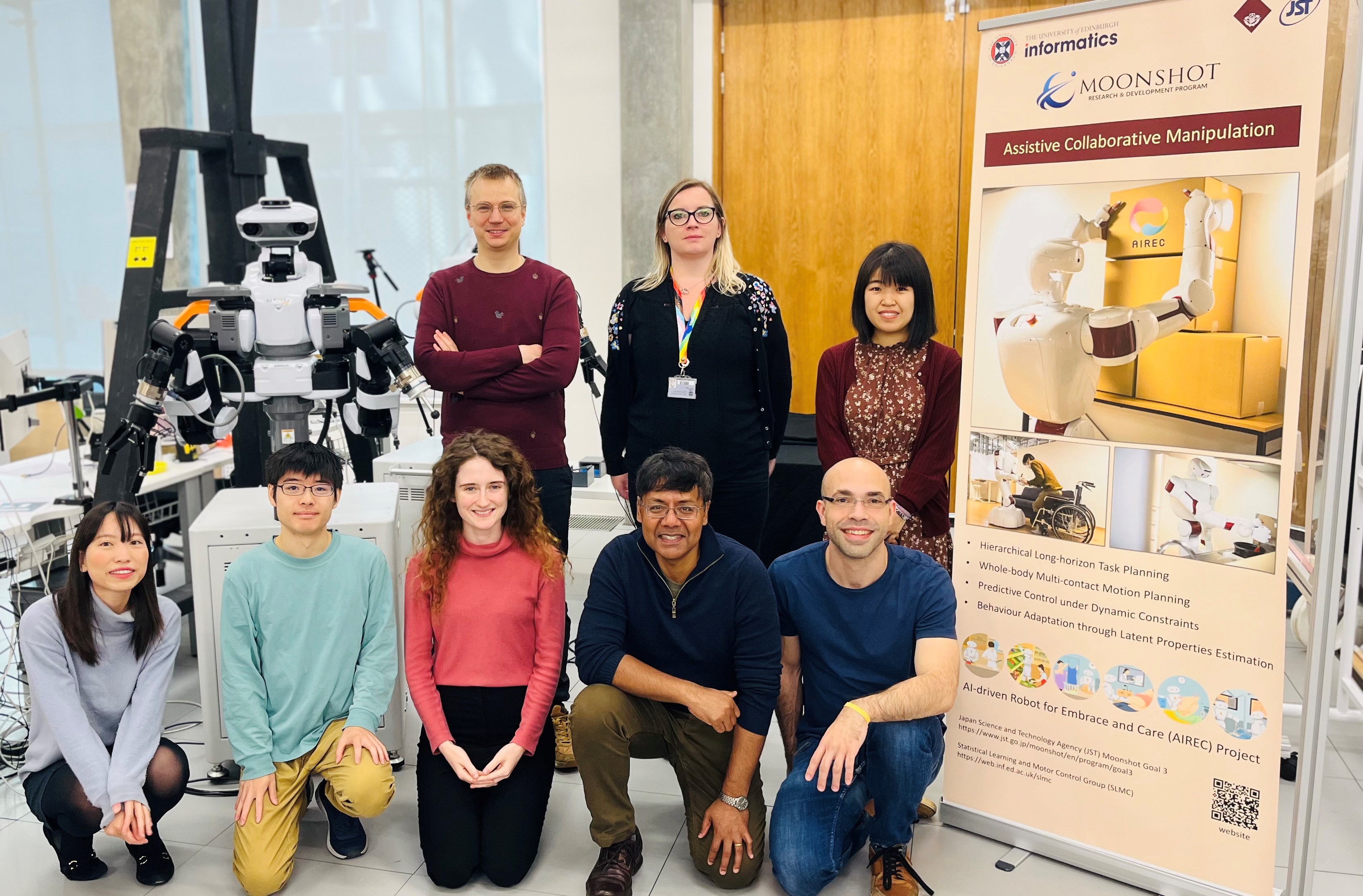
Please check out below our results and events organised as part of the Moonshot programme (in reverse chronological order):